Factors Associated with Caesarean Delivery in Nigeria: a Generalized Linear Mixed Logistic Regression Analysis Using Adaptive Gaussian Quadrature Technique
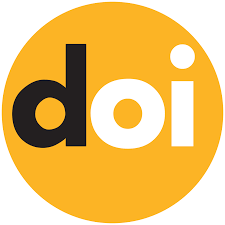
Keywords:
Caesarean births, Adaptive Gaussian Quadrature, Generalized logistic mixed modelAbstract
Background: There has been an increase in the prevalence of caesarean births in recent decades, a development that has assumed a public health issue of global concern. An analytical technique that reduces loss of information in caesarean section modeling by accounting for grouping effect is of great need. This study assessed the correlates of caesarean deliveries in Nigeria using a generalized logistic mixed model and adopted the Adaptive Gaussian Quadrature estimation techniques.
Methods: The study extracted data on women of childbearing age from the Nigerian Demographic and Health Survey (NDHS) 2013. Generalized logistic mixed model was used to assess the correlates of caesarean deliveries. Data was analyzed using SPSS version 23.
Results: The overall prevalence of caesarean section delivery is 6.8% and in keeping with the WHO recommendation for caesarean section birth rates. The predictors of caesarean section were history of caesarean section, ever having a termination of pregnancy, maternal age, birth order, if current pregnancy is a twin, size of the baby and distance of clients’ residence to the health facility. The prevalence of caesarean section births was higher among mothers living in urban communities, mothers with higher educational attainments and those with high ranks in the wealth index. The covariates found to significantly influence caesarean births in this study are in line with WHO recommendations
Conclusion: Wide variations exist in the predictors of caesarean section births, and this calls for a region-specific programme design aimed at ensuring rational indications for caesarean section delivery, hence avoiding preventable caesarean section.
Downloads
Published
How to Cite
Issue
Section
License
Copyright (c) 2022 OA Okunlola, WO Adebimpe, DO Ibirongbe, OO Osunmakinwa, O Awe, S Adetokunbo, AF Lukman

This work is licensed under a Creative Commons Attribution-NonCommercial-ShareAlike 4.0 International License.